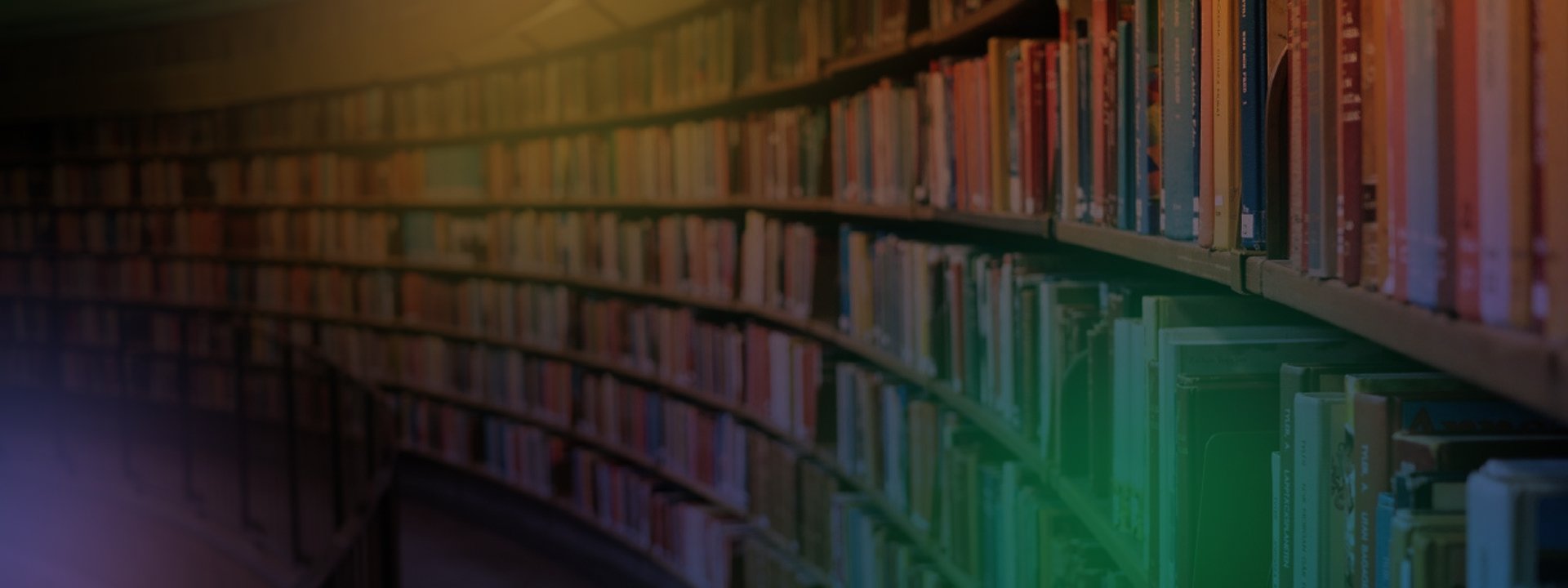
Most cited publications
A vision transformer for decoding surgeon activity from surgical videos
Dani Kiyasseh, Runzhuo Ma, Taseen F Haque, Brian J Miles, Christian Wagner, Daniel A Donoho, Animashree Anandkumar, Andrew J Hung
Publication date: 2023/6
Journal: Nature Biomedical Engineering
The intraoperative activity of a surgeon has substantial impact on postoperative outcomes. However, for most surgical procedures, the details of intraoperative surgical actions, which can vary widely, are not well understood. Here we report a machine learning system leveraging a vision transformer and supervised contrastive learning for the decoding of elements of intraoperative surgical activity from videos commonly collected during robotic surgeries. The system accurately identified surgical steps, actions performed by the surgeon, the quality of these actions and the relative contribution of individual video frames to the decoding of the actions. Through extensive testing on data from three different hospitals located in two different continents, we show that the system generalizes across videos, surgeons, hospitals and surgical procedures, and that it can provide information on surgical gestures and skills from …
Development and clinical validation of a grading system for pituitary adenoma consistency
Martin J Rutkowski, Ki-Eun Chang, Tyler Cardinal, Robin Du, Ali R Tafreshi, Daniel A Donoho, Andrew Brunswick, Alexander Micko, Chia-Shang J Liu, Mark S Shiroishi, John D Carmichael, Gabriel Zada
Publication date: 2020/6/5
Journal: Journal of neurosurgery
OBJECTIVE
Pituitary adenoma (PA) consistency, or texture, is an important intraoperative characteristic that may dictate operative dissection techniques and/or instruments used for tumor removal during endoscopic endonasal approaches (EEAs). The impact of PA consistency on surgical outcomes has yet to be elucidated.
METHODS
The authors developed an objective 5-point grading scale for PA consistency based on intraoperative characteristics, including ease of tumor debulking, manipulation, and instrument selection, ranging from cystic/hemorrhagic tumors (grade 1) to calcified tumors (grade 5). The proposed grading system was prospectively assessed in 306 consecutive patients who underwent an EEA for PAs, and who were subsequently analyzed for associations with surgical outcomes, including extent of resection (EOR) and complication profiles.
RESULTS
Institutional database review identified 306 …
A systematic review of virtual reality for the assessment of technical skills in neurosurgery
Justin Chan, Dhiraj J Pangal, Tyler Cardinal, Guillaume Kugener, Yichao Zhu, Arman Roshannai, Nicholas Markarian, Aditya Sinha, Anima Anandkumar, Andrew Hung, Gabriel Zada, Daniel A Donoho
Publication date: 2021/8/1
Journal: Neurosurgical Focus
OBJECTIVE
Virtual reality (VR) and augmented reality (AR) systems are increasingly available to neurosurgeons. These systems may provide opportunities for technical rehearsal and assessments of surgeon performance. The assessment of neurosurgeon skill in VR and AR environments and the validity of VR and AR feedback has not been systematically reviewed.
METHODS
A systematic review following the Preferred Reporting Items for Systematic Reviews and Meta-Analyses (PRISMA) guidelines was conducted through MEDLINE and PubMed. Studies published in English between January 1990 and February 2021 describing the use of VR or AR to quantify surgical technical performance of neurosurgeons without the use of human raters were included. The types and categories of automated performance metrics (APMs) from each of these studies were recorded …
A multi-institutional study using artificial intelligence to provide reliable and fair feedback to surgeons
Dani Kiyasseh, Jasper Laca, Taseen F Haque, Brian J Miles, Christian Wagner, Daniel A Donoho, Animashree Anandkumar, Andrew J Hung
Publication date: 2023/3/30
Journal: Communications Medicine
Background
Surgeons who receive reliable feedback on their performance quickly master the skills necessary for surgery. Such performance-based feedback can be provided by a recently-developed artificial intelligence (AI) system that assesses a surgeon’s skills based on a surgical video while simultaneously highlighting aspects of the video most pertinent to the assessment. However, it remains an open question whether these highlights, or explanations, are equally reliable for all surgeons.
Methods
Here, we systematically quantify the reliability of AI-based explanations on surgical videos from three hospitals across two continents by comparing them to explanations generated by humans experts. To improve the reliability of AI-based explanations, we propose the strategy of training with explanations –TWIX –which uses human explanations as supervision to explicitly teach an AI system to highlight important …
Robotic and robot-assisted skull base neurosurgery: systematic review of current applications and future directions
Dhiraj J Pangal, David J Cote, Jacob Ruzevick, Benjamin Yarovinsky, Guillaume Kugener, Bozena Wrobel, Elisabeth H Ference, Mark Swanson, Andrew J Hung, Daniel A Donoho, Steven Giannotta, Gabriel Zada
Publication date: 2022/1/1
Journal: Neurosurgical Focus
OBJECTIVE
The utility of robotic instrumentation is expanding in neurosurgery. Despite this, successful examples of robotic implementation for endoscopic endonasal or skull base neurosurgery remain limited. Therefore, the authors performed a systematic review of the literature to identify all articles that used robotic systems to access the sella or anterior, middle, or posterior cranial fossae.
METHODS
A systematic review of MEDLINE and PubMed in accordance with PRISMA guidelines performed for articles published between January 1, 1990, and August 1, 2021, was conducted to identify all robotic systems (autonomous, semiautonomous, or surgeon-controlled) used for skull base neurosurgical procedures. Cadaveric and human clinical studies were included. Studies with exclusively otorhinolaryngological applications or using robotic microscopes were excluded …
A guide to annotation of neurosurgical intraoperative video for machine learning analysis and computer vision
Dhiraj J Pangal, Guillaume Kugener, Shane Shahrestani, Frank Attenello, Gabriel Zada, Daniel A Donoho
Publication date: 2021/6/1
Journal: World neurosurgery
Objective
Computer vision (CV) is a subset of artificial intelligence that performs computations on image or video data, permitting the quantitative analysis of visual information. Common CV tasks that may be relevant to surgeons include image classification, object detection and tracking, and extraction of higher order features. Despite the potential applications of CV to intraoperative video, however, few surgeons describe the use of CV. A primary roadblock in implementing CV is the lack of a clear workflow to create an intraoperative video dataset to which CV can be applied. We report general principles for creating usable surgical video datasets and the result of their applications.
Methods
Video annotations from cadaveric endoscopic endonasal skull base simulations (n = 20 trials of 1–5 minutes, size = 8 GB) were reviewed by 2 researcher-annotators. An internal, retrospective analysis of workflow for development …
Costs and training results of an objectively validated cadaveric perfusion‐based internal carotid artery injury simulation during endoscopic skull base surgery
Daniel A Donoho, Cali E Johnson, Kevin T Hur, Ian A Buchanan, Vance L Fredrickson, Michael Minneti, Gabriel Zada, Bozena B Wrobel
Publication date: 2019/7
Journal: International Forum of Allergy & Rhinology
Background Internal carotid artery injury (ICAI) is a rare, life‐threatening complication of endoscopic endonasal approaches (EEAs). High‐fidelity simulation methods exist, but optimization of the training cohort, training paradigm, and costs of simulation training remain unknown. Methods Using our previously validated, high‐fidelity, perfused‐cadaver model, participants attempted to manage a simulated ICAI. After a brief instructional video and coaching, the simulation was repeated. Training success was defined as successful ICAI control on the second attempt after failure on the initial attempt. Marginal costs were measured. Results Seventy‐two surgeons participated in the standardized simulation, which lasted≤ 15 minutes. The marginal cost of simulation was 275.00persurgeon.Atotalof44.4%(n=32)succeededonthefirstattemptbeforetraining(previouslyproficient);44.4%(n=32)failedthefirstattempt …
Expert surgeons and deep learning models can predict the outcome of surgical hemorrhage from 1 min of video
Dhiraj J Pangal, Guillaume Kugener, Yichao Zhu, Aditya Sinha, Vyom Unadkat, David J Cote, Ben Strickland, Martin Rutkowski, Andrew Hung, Animashree Anandkumar, XY Han, Vardan Papyan, Bozena Wrobel, Gabriel Zada, Daniel A Donoho
Publication date: 2022/5/17
Journal: Scientific reports
Major vascular injury resulting in uncontrolled bleeding is a catastrophic and often fatal complication of minimally invasive surgery. At the outset of these events, surgeons do not know how much blood will be lost or whether they will successfully control the hemorrhage (achieve hemostasis). We evaluate the ability of a deep learning neural network (DNN) to predict hemostasis control ability using the first minute of surgical video and compare model performance with human experts viewing the same video. The publicly available SOCAL dataset contains 147 videos of attending and resident surgeons managing hemorrhage in a validated, high-fidelity cadaveric simulator. Videos are labeled with outcome and blood loss (mL). The first minute of 20 videos was shown to four, blinded, fellowship trained skull-base neurosurgery instructors, and to SOCALNet (a DNN trained on SOCAL videos). SOCALNet architecture …
Use of surgical video–based automated performance metrics to predict blood loss and success of simulated vascular injury control in neurosurgery: a pilot study
Dhiraj J Pangal, Guillaume Kugener, Tyler Cardinal, Elizabeth Lechtholz-Zey, Casey Collet, Sasha Lasky, Shivani Sundaram, Yichao Zhu, Arman Roshannai, Justin Chan, Aditya Sinha, Andrew J Hung, Animashree Anandkumar, Gabriel Zada, Daniel A Donoho
Publication date: 2021/12/31
Journal: Journal of Neurosurgery
OBJECTIVE
Experts can assess surgeon skill using surgical video, but a limited number of expert surgeons are available. Automated performance metrics (APMs) are a promising alternative but have not been created from operative videos in neurosurgery to date. The authors aimed to evaluate whether video-based APMs can predict task success and blood loss during endonasal endoscopic surgery in a validated cadaveric simulator of vascular injury of the internal carotid artery.
METHODS
Videos of cadaveric simulation trials by 73 neurosurgeons and otorhinolaryngologists were analyzed and manually annotated with bounding boxes to identify the surgical instruments in the frame. APMs in five domains were defined—instrument usage, time-to-phase, instrument disappearance, instrument movement, and instrument interactions—on the basis of expert analysis and task …
Potential applications of artificial intelligence and machine learning in spine surgery across the continuum of care
Samuel R Browd, Christine Park, Daniel A Donoho
Publication date: 2023/6/1
Journal: International Journal of Spine Surgery
The worlds of spinal surgery and computational science are intersecting at the nexus of the operating room and across the continuum of patient care. As medicine moves toward digitizing all aspects of a patient’s care, immense amounts of patient data generated and aggregated across surgeons, procedures, and institutions will enable previously inaccessible computationally driven insights. These early insights from artificial intelligence (AI) and machine learning (ML)–enabled technologies are beginning to transform medicine and surgery. The complex pathologies facing spine surgeons and their patients require integrative, multimodal, data-driven management strategies. As these data and the technological tools to computationally process them become increasingly available to spine surgeons, AI and ML methods will inform patient selection, preoperatively risk-stratify patients based on myriad factors, and inform …
Validation of Machine Learning–Based Automated Surgical Instrument Annotation Using Publicly Available Intraoperative Video
Nicholas Markarian, Guillaume Kugener, Dhiraj J Pangal, Vyom Unadkat, Aditya Sinha, Yichao Zhu, Arman Roshannai, Justin Chan, Andrew J Hung, Bozena B Wrobel, Animashree Anandkumar, Gabriel Zada, Daniel A Donoho
Publication date: 2022/9/1
Journal: Operative Neurosurgery
BACKGROUND:
Intraoperative tool movement data have been demonstrated to be clinically useful in quantifying surgical performance. However, collecting this information from intraoperative video requires laborious hand annotation. The ability to automatically annotate tools in surgical video would advance surgical data science by eliminating a time-intensive step in research.
OBJECTIVE:
To identify whether machine learning (ML) can automatically identify surgical instruments contained within neurosurgical video.
METHODS:
A ML model which automatically identifies surgical instruments in frame was developed and trained on multiple publicly available surgical video data sets with instrument location annotations. A total of 39 693 frames from 4 data sets were used (endoscopic endonasal surgery [EEA][30 015 frames], cataract surgery [4670], laparoscopic cholecystectomy [2532], and microscope-assisted brain …
Deep neural networks can accurately detect blood loss and hemorrhage control task success from video
Guillaume Kugener, Yichao Zhu, Dhiraj J Pangal, Aditya Sinha, Nicholas Markarian, Arman Roshannai, Justin Chan, Animashree Anandkumar, Andrew J Hung, Bozena B Wrobel, Gabriel Zada, Daniel A Donoho
Publication date: 2022/6/1
Journal: Neurosurgery
BACKGROUND:
Deep neural networks (DNNs) have not been proven to detect blood loss (BL) or predict surgeon performance from video.
OBJECTIVE:
To train a DNN using video from cadaveric training exercises of surgeons controlling simulated internal carotid hemorrhage to predict clinically relevant outcomes.
METHODS:
Video was input as a series of images; deep learning networks were developed, which predicted BL and task success from images alone (automated model) and images plus human-labeled instrument annotations (semiautomated model). These models were compared against 2 reference models, which used average BL across all trials as its prediction (control 1) and a linear regression with time to hemostasis (a metric with known association with BL) as input (control 2). The root-mean-square error (RMSE) and correlation coefficients were used to compare the models; lower RMSE indicates …